As the days get shorter in winter, it is almost impossible to avoid traveling in the dark. This is a particular problem for drivers: at night, especially on monotonous routes like highways, fatigue symptoms such as drowsiness and inattentiveness, sometimes even brief nodding off, occur. These phenomena obviously pose an immense risk, for the person driving the vehicle as well as for third parties in the vehicle or other uninvolved road users and pedestrians. The significant number of accidents which can be attributed to fatigue is a motivation for research and the search for solutions.
Professor Martin Golz from the Faculty of Computer Science at Schmalkalden University of Applied Sciences addresses these questions from the research field of biomedical engineering: In order to measure a phenomenon, its definition has to be clear. The first question is therefore what vigilance is and, in turn, in what forms symptoms of fatigue occur.[1] It is important to differentiate between various aspects and at the same time to identify the decisive factors. The aim is therefore to find one or more reliable criteria that can be used to detect signs of fatigue. A central challenge for research is that there is no binding definition of fatigue or its signs and, as a result, there is a lack of reliable and decisive attributes.[2] So how do we measure the characteristics of fatigue?
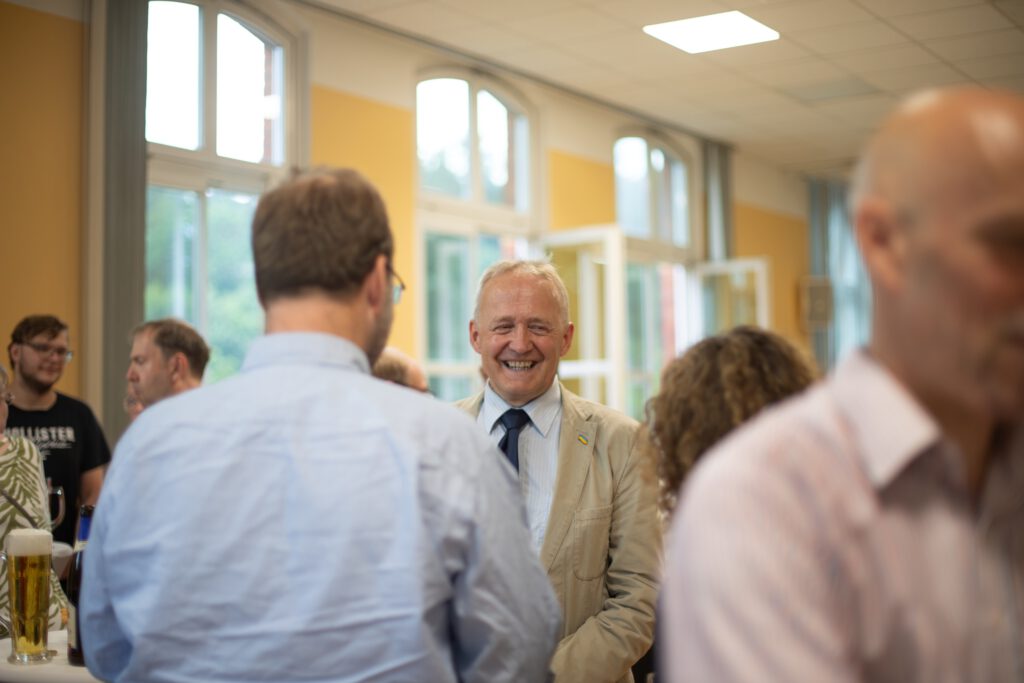
What is fatigue and how does it manifest itself?
An opportunity to explore this question arose as part of an inquiry from industry, specifically the open-cast mining industry: in mining operations, there is a lot of logistics using huge trucks that work in shifts.[3] Several factors that usually cause fatigue come together here: Long journeys, monotonous routes, constant repetition. The strain is further increased by the harsh environmental conditions such as high temperatures, low humidity and dust. In short: a back-breaking job whose high physical and mental strain regularly leads to accidents due to fatigue. Political and economic players have a vested interest in preventing these accidents due to the physical and economic damage and consequential costs.
In recent decades, industry has undergone a rethink that has focused attention on employee fitness: fatigue risk management has introduced structures and ways of dealing with fatigue into the workplace that help to deal with symptoms of fatigue openly and consciously, recognize them at an early stage and thus prevent accidents. Furthermore, supervisors should draw attention to the phenomenon and the risk and raise awareness among the workforce. In order to further promote the safety of drivers, monitoring technologies that autonomously detect signs of fatigue and sleep events and, at best, reliably alert before accidents occur are useful and expedient. Two things come together here: Firstly, measuring the manifestations of fatigue, and secondly, a prognosis. Both points must be pursued in research using different approaches.
Monitoring systems
In order to be able to evaluate the use of the monitoring systems, first a selection had to be made from the systems available on the market. In addition to scientists, employees and company representatives were also consulted: The weighting of various factors resulted in a coordinate system that allowed three monitoring models to be identified as useful test cases. These three have a similar technical design: they are mounted on or in the dashboard and are equipped with an infrared camera.
They measure fatigue using the PERCLOS scale: this refers to the time while the eye is at least eighty percent covered by the eyelid. For this measurement, the camera records various aspects, which it only evaluates in combination with PERCLOS. In addition to the opening of the eyelids, the duration of eyelid closure and pupil width are included in the evaluation, as is head movement, among other things. In addition to the significance of the PERCLOS criterion itself, it is unclear whether one or more aspects alone provide valid information about fatigue. For the monitoring, it is important to use a high level of sensitivity on the one hand, but on the other to avoid false alarms as far as possible, as this in turn would reduce acceptance. For example, closing your eyes when oncoming traffic is dazzling must not lead to an alarm. In this consideration, a feasible method of application must be found.
Test drives in the lab
Due to the risk involved, tests in real-life situations are not possible. As a result, Professor Golz use a computer-aided driving simulation, which allows the measurement of fatigue symptoms under controlled conditions. The first task was to find the test subjects: Several suitable people were selected from a large number of applications from the student body at Schmalkalden University of Applied Sciences to take part in the test. In order to make fatigue more likely – the aim was to measure it – the participants had to adhere to a certain day/night rhythm, keep a sleep log, refrain from napping during the day and avoid drinks that keep them awake, such as coffee, on the day of the measurement. Simulated test drives were modeled on the real work routines of the drivers in the opencast mine. From 23:30 to 08:30, the sixteen participants had to drive several forty-minute units. The test environment and test conditions were designed to promote fatigue: The route was monotonous, there was no road traffic and no distractions during the ride. In summary, the important criteria of the study were to make symptoms of fatigue more likely: Time since sleep, time on task, time of day and the perception of monotony.
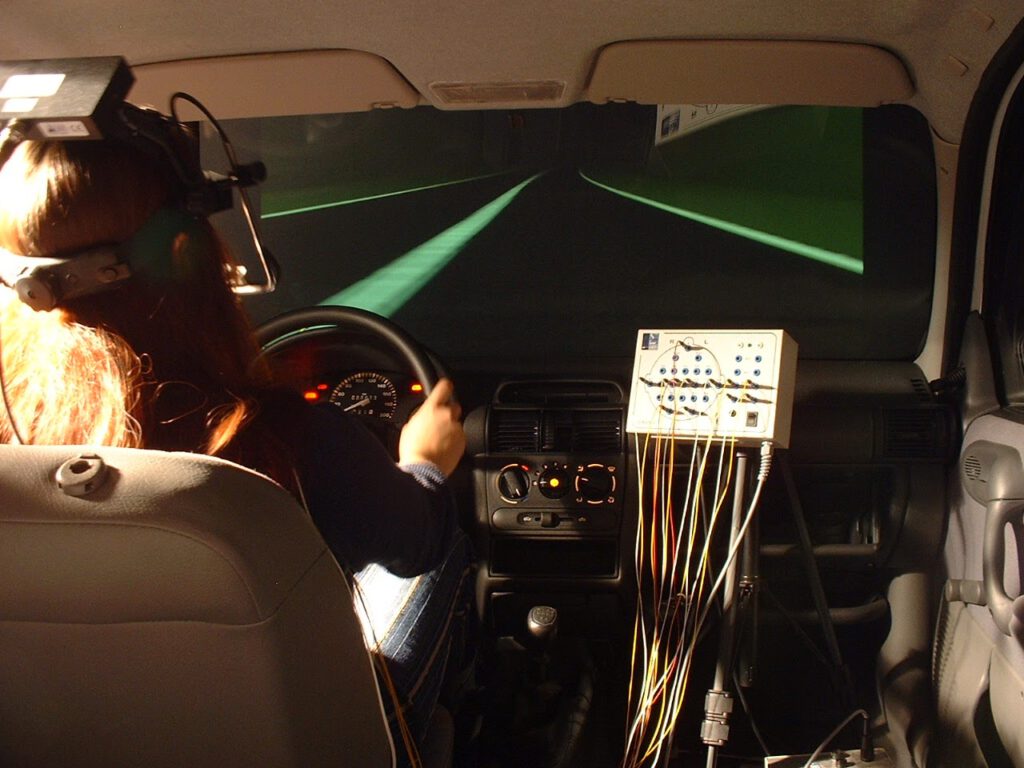
Two established scales were used as a reference for determining tiredness, which function independently of the other values and could therefore serve as control variables: Firstly, the self-assessment of the degree of momentary fatigue, which was queried in the breaks between rides (Karolinska sleepiness scale). This nine-point scale has proven to be quite reliable in science for measuring subjectively perceived fatigue. Secondly, the position of the vehicle on the road: effects of fatigue cause vehicles to leave the center of the road more frequently. Crossing lane markings can be easily determined. Both criteria should form the basis for evaluating the monitoring systems. Professor Golz chose EOG and EEG signals, i.e. eye and brain activity, as a further source of measurement.
We are mostly familiar with EEG images from television: A network of cables is placed over a head and contact is made with the scalp at various locations. These contacts can be used to measure the slightest potentials of an electrical current field and thus to trace the activity in the brain to a certain extent. Depending on the number of contacts, recordings of a large number of waves are created that are meaningless to the layman. The evaluation of these signals is a highly complex matter and requires a great deal of experience. Only in this way can differentiated conclusions be drawn from the shifts in the waves, their frequencies and amplitudes and depending on the area of the brain. One question is whether signs of fatigue can be precisely determined using this method, or whether it is possible to predict the onset of fatigue.
Collection and evaluation of data
In a first step, the data from the two independent measurements were compared with the PERCLOS surveys of the three monitoring systems. The correlation of the PERCLOS values with the objective measurements was only superficial: as soon as the temporal resolution becomes finer or only the data of one individual is used, the high correlation values are lost. In summary, significant inter- and even intrasubjective differences were found across the scales.
In a second step, Professor Golz attempted to clarify whether the EEG measurements could serve as a reference for validating the video-based systems. In order to be able to carry out a data analysis, both measurements were collected simultaneously and the data sets were then divided into strong and weak manifestations of fatigue using the independent variables. A non-linear discriminant analysis of both the PERCLOS and the EEG/EOG time series showed a deficit of PERCLOS in distinguishing between weak and strong fatigue in high temporal resolution.
From these results, it can be concluded that although the monitoring systems work well under laboratory conditions, important conditions must be met for this: In addition to a low temporal resolution, the data must be averaged over as many people as possible before evaluation. It remains to be seen whether the measurement deficits are due to the alignment of the systems with the normal behavior of eye movement, as a result of which atypical characteristics would not be detected or would be detected incorrectly. In any case, the EEG/EOG signal proved to be a much more robust measure for determining fatigue.
In the end, it can be said that there is still a long way to go to achieve functioning monitoring systems for signs of fatigue. One problem is that there is still no clarity as to how fatigue manifests itself, i.e. what are generalizable criteria and measurement objectives. PERCLOS has only been able to convince to a very limited extent. And since this area is literally a matter of life and death, more research is needed to provide suitable benchmarks for monitoring approaches.
The prediction of microsleep events
Even if the EEG can reliably detect signs of fatigue, the practical problem remains that determining the data is time-consuming and requires expert knowledge. Other technological solutions must be found in order to achieve broad acceptance. The research field of neuroinformatics therefore still has plenty of work to do here. Predictive analytics in particular, i.e. the prediction of critical signs of fatigue, has social and economic relevance.
In another, more recent project, Professor Golz and his team focused on the predictability of microsleep events with the aid of EEG signals.[4] The challenges again are laying in the complexity of the signal characteristics and the high inter-individual variability, i.e. different EEG properties depending on the person. The idea was to analyze the amplitudes of the EEG using five methods of time series prediction. In addition to the predictive quality, it was also about the suitability of different EEG measurement points, i.e. which brain area is more or less suitable for the measurements.
The data came from various test drives in the Schmalkalden laboratory, which were carried out under the same conditions as the fatigue survey. By using the measured biosignals from 79 young, healthy people, it was possible to draw on a large amount of data. Even though one model of the time series prediction was characterized by a low number of errors, it became clear that each EEG channel required different parameter combinations for its optimal use.
This complexity makes predictions difficult, especially when the time horizon is set at five seconds. Even an adaptive classification analysis, which should be able to cope with the dynamic character of the signals, had high accuracies in the first step, but was unable to reproduce these results in the validation. In conclusion, the performance of the prediction is high for most individuals, but for some it is still quite low. To enable improvements here, optimized data sets could be used as well as modern methods that make use of artificial intelligence and correspond to the dynamic nature of the data. Ultimately, any improvement in predictability can help save lives. It is therefore important to continue research into how fatigue can be recognised. The driving simulation laboratory and the archive of data records obtained are an ideal starting point for this.
[1] Vigilance comes from the Latin vigilantia, which means “alertness” and “care”. Vigilance refers to a state of sustained attention with a monotonous stimulus frequency and is colloquially synonymous with alertness, an aspect of consciousness. Vigilance is measured by examining a person’s ability to pay sustained attention.
[2] The ambiguous use of the terms tiredness, fatigue and exhaustion is part of the problem. Researchers are now arguing in favor of defining the three terms as distinct phenomena on a continuum that can be placed in relation to each other. See Matti, N., Mauczok, C. & Specht, M.B. (2022): Müdigkeit, Fatigue und Erschöpfung: Alles das Gleiche oder Ausprägungen eines Kontinuums? – Ein Diskussionsanstoß. Somnologie – Schlafforschung und Schlafmedizin 26, 187-198.
[3] Golz, M., Sommer, D., Trutschel, U., Sirois, B., Edwards, D. (2010): Evaluation of fatigue monitoring technologies. Somnologie – Schlafforschung und Schlafmedizin 14, 187-199.
Schneeweiß, L., Pauli, M. P., Golz, M. (2023): EEG-Vorhersage zur Prognose von Mikroschlaf, in: Stolzenburg, F., Reinboth, C., Lohr, T. & Vogel, K. (Hrsg.): NWK 2023 – Tagungsband der 23. Nachwuchswissenschaftler*innenkonferenz, Harzer Hochschultexte Nr. 14, Hochschule Harz, Wernigerode, 176-183.